Unveiling the Secrets of Profitable Cryptocurrency Arbitrage: A Comprehensive Guide
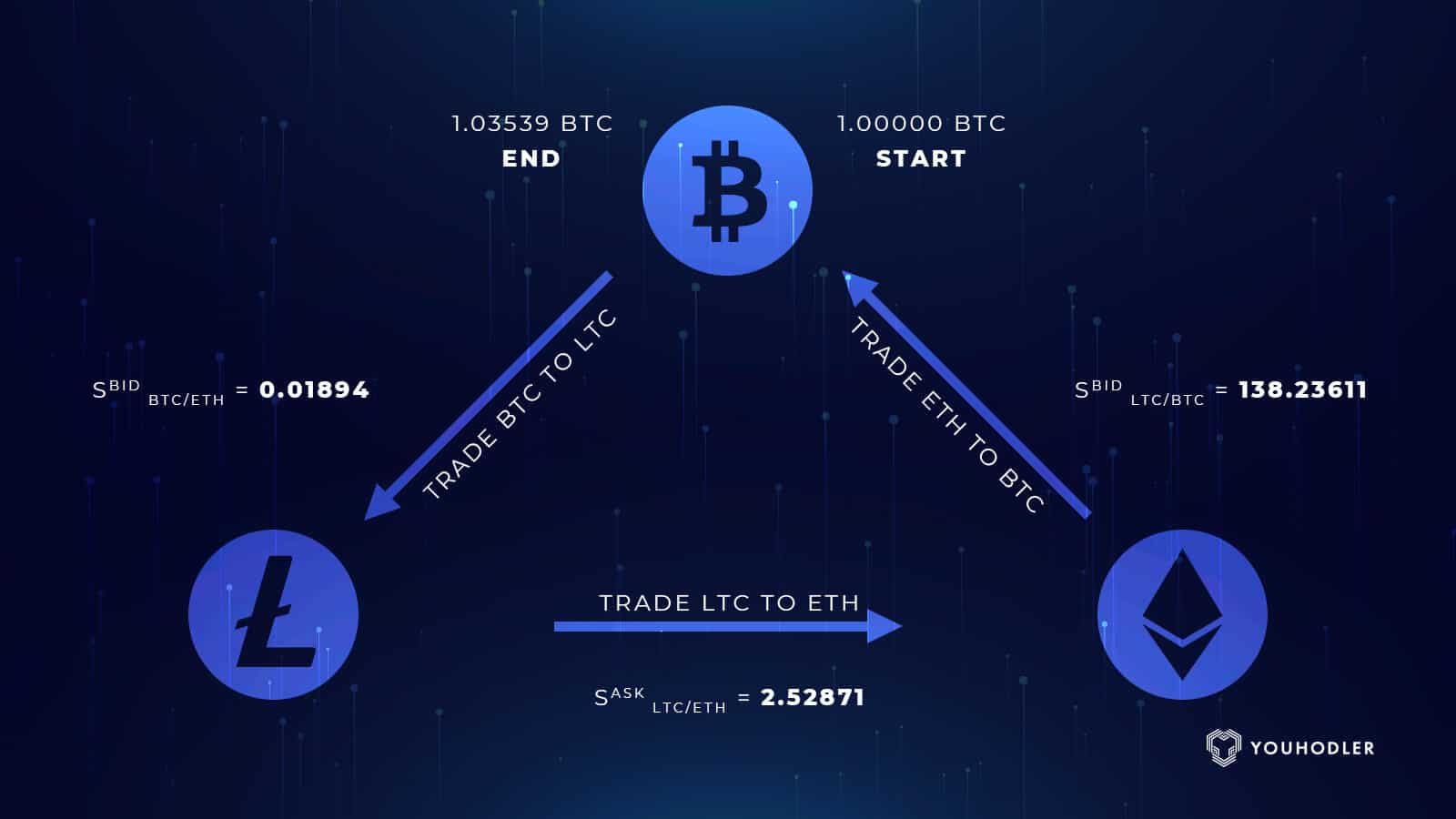
Lately, there has been a surge in discussions about “arbitrage” and how individuals are engaging in it, planning to do so, or boasting about their incredible profits from arbitraging cryptocurrencies using self-programmed “bots” with instructions found on YouTube. Surprisingly, there are even initial coin offerings (ICOs) that have raised funds for these activities without adequately addressing key aspects of arbitrage and lacking teams with the necessary domain expertise.
These conversations have taken place among programmers in prominent tech hubs like Silicon Valley, Hong Kong, and New York. Having knowledge and experience in arbitrage, high yield trading, and financial engineering, we decided to share our insights in this article. We aim to clarify what arbitrage truly entails, explore its various forms, and shed light on the existing opportunities within the realm of cryptocurrencies.
To cut to the chase, there were indeed numerous opportunities for cryptocurrency “deterministic arbitrage” at least until late 2017. Although those opportunities have diminished, there are still possibilities in “statistical arbitrage,” as well as a few intriguing ones in “regulatory arbitrage.” However, the most captivating area lies within what we refer to as “hashing arbitrage.” It incorporates elements from all the previously mentioned forms but possesses a unique essence of its own.
As we mentioned earlier, there are still existing opportunities within the crypto space. However, those who are knowledgeable about them prefer not to openly discuss, publish papers, or release their code as open source or through platforms like Kaggle kernel competitions. Sharing knowledge in the crypto world differs vastly between Wall Street and Silicon Valley, with the latter being far less inclined to do so.
According to Merriam-Webster, arbitrage is defined as:
“The nearly simultaneous purchase and sale of securities or foreign exchange in different markets in order to profit from price discrepancies.”
In this article, we won’t delve into the details of “hashing arbitrage” or regulatory arbitrage. Instead, we’ll quote the definition of regulatory arbitrage from Investopedia, as it provides a comprehensive explanation:
“Regulatory arbitrage is a practice whereby firms capitalize on loopholes in regulatory systems in order to circumvent unfavorable regulation. Arbitrage opportunities may be accomplished by a variety of tactics, including restructuring transactions, financial engineering, and geographic relocation. While it’s difficult to entirely prevent regulatory arbitrage, its prevalence can be limited by closing the most evident loopholes and increasing the costs associated with circumventing regulations.”
Before we discuss the distinctions between deterministic and statistical arbitrage, and clarify which type of arbitrage most cryptocurrency traders are referring to, it’s crucial to address the concept of “Market Making.”
When participants wish to buy or sell a financial product, they must navigate their way to an exchange where buyers and sellers convene. The price at which they can trade depends on the current supply and demand of the product, which is reflected in the bid price for purchasing and the ask price for selling. If there is a limited number of counterparties to trade with, it may become difficult or even impossible to buy or sell the product, rendering it illiquid.
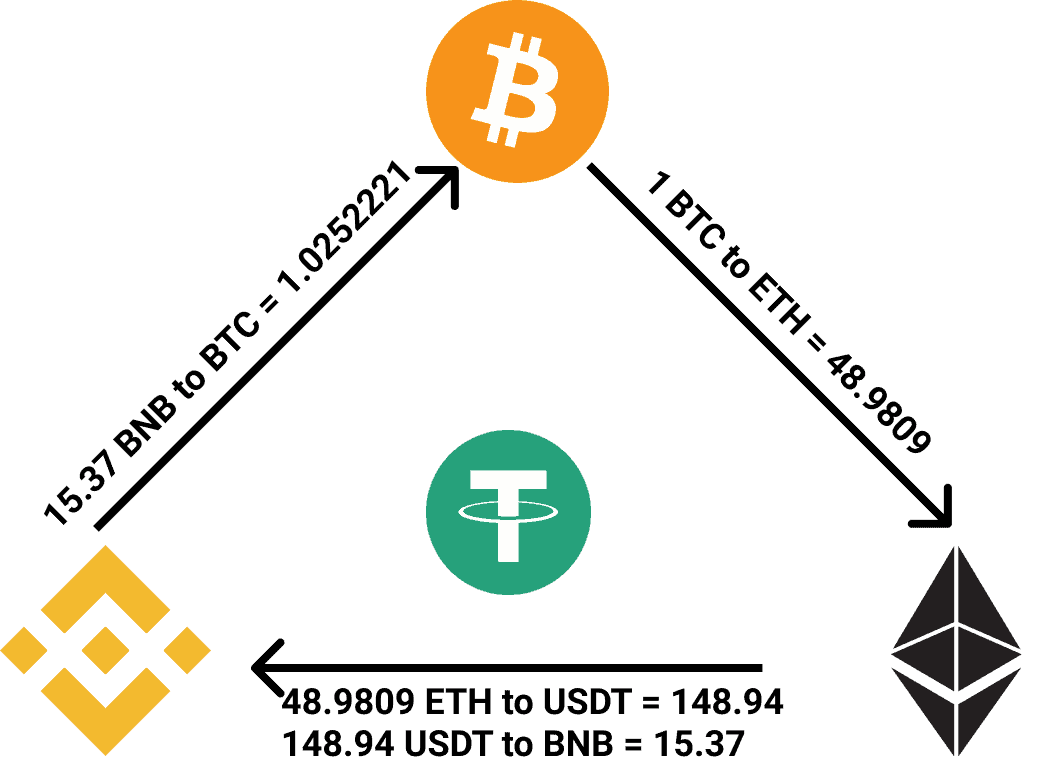
In order to ensure sufficient liquidity, exchanges rely on professionals known as market makers who continuously provide bid-ask spreads to the market. These market makers are responsible for creating a market, hence the term “market makers.” Their objective is not to predict the price movement of a product but to profit from the difference between the bid and ask prices, also known as the spread.
When a market maker trades on either side of the spread, they take on a position in the market, which introduces a certain level of risk. To mitigate this risk, market makers employ various strategies such as hedging their positions with different products. Consequently, market makers need to possess a deep understanding of the product they are making markets in, as well as its relationship with other similar financial products.
Over time, competition and advancements in technology have significantly transformed the role of market makers. To provide competitive quotes across multiple exchanges and products, market makers now rely on trading algorithms and electronic exchange connectivity facilitated by computer systems. Major investment banks like JPMorgan, Morgan Stanley, and Goldman Sachs dominate this financial activity. However, their presence in the cryptocurrency markets remains limited due to regulatory constraints.
To stay competitive and contribute to efficient financial markets, market makers must continuously invest in technology and skilled professionals. These advancements and increased competition have made the job of market makers considerably more complex. By tightening the bid-ask spread, market makers provide tangible benefits by reducing transaction costs associated with buying or selling securities.
Now, armed with a basic understanding of liquidity and the influence of technology, we can differentiate between two main types of arbitrage: deterministic arbitrage and statistical arbitrage.
Deterministic arbitrage occurs when an investor simultaneously buys and sells an asset to profit from an existing price difference on similar or identical securities. This arbitrage technique enables investors to regulate the market and help minimize price discrepancies, ensuring that securities trade at fair market values.
However, due to technological advancements in trading traditional securities, profiting from pricing inefficiencies in the market has become extremely challenging. Leading financial institutions heavily invest in IT infrastructure and computerized trading systems to monitor fluctuations in similar financial instruments. Any opportunities arising from inefficient pricing are swiftly seized, often within seconds.
Technology has always provided an edge to those who possess it, as demonstrated by the visionary individuals who capitalized on price differentials between stock exchanges in California and New York during the early 20th century. Back then, those who had access to cutting-edge technologies such as private telephones and telegraphs could obtain price information for certain railroad stocks in California and New York during times of volatility, enabling them to execute risk-free transactions through their brokers.
Arbitrage in the cryptocurrency market follows a similar pattern to that of legacy securities. Until late 2017, there was virtually no institutional presence in the cryptocurrency asset class. If you possessed knowledge of Python, basic data analysis skills, and a rudimentary understanding of finance, you could have potentially made some money through what you might label as “deterministic arbitrage.”
We established an “Arb Event” as a time-based function and utilized the data we gathered to compile a table. Through our analysis, we discovered that the most significant arbitrage opportunities in terms of both frequency and value occurred last year in the BTC-USD/USDT and BTC-ETH trading pairs.
During that specific period, our analysis revealed that the average duration of an arbitrage opportunity was approximately 9 minutes, accompanied by an average profit margin of around 5%. Notably, the exchanges Exmo and OKCoin constituted almost two-thirds of all the arbitrage opportunities observed.
So, let’s delve into the concept of “statistical arbitrage.”
b) Statistical arbitrage is an extensively quantitative and computational approach to trading, incorporating data mining, statistical methods, and automated trading systems. In this domain, we find hedge fund firms like Quantbot Technologies, Bridgewater Associates (managing $150 billion USD), and 2 Sigma as prominent players. These firms heavily invest in technology and recruit top-notch quants from Wall Street, while also training programmers and computer scientists from Silicon Valley (who may have limited exposure to time series analysis or finance expertise) to think like quants. However, their involvement in cryptocurrencies remains minimal or insignificant.
Historically, statistical arbitrage has emerged from pairs trading strategies, where stocks are paired based on fundamental or market-based similarities. When one stock outperforms the other, the underperforming stock is bought long with the expectation that it will rise toward its outperforming counterpart, while the other stock is sold short. Mathematically speaking, the strategy revolves around identifying asset pairs with high cointegration.
In statistical arbitrage, portfolio construction entails two phases. First, the scoring phase assigns a numeric score or rank to each asset in the market, reflecting its desirability—similar to Google’s page rank, but for financial assets. The scoring process is intuitive, with high scores indicating “go long” and low scores indicating “go short.”
The second phase involves risk reduction, where assets are combined in carefully matched proportions to eliminate risks. However, one must remain aware of these risks, and this is where casual crypto traders often stumble.
The scoring aspect within quant shops and hedge funds is fascinating and highly proprietary. The details of the scoring formula vary and are closely guarded. I have developed my own scoring system, which we have successfully applied to cryptocurrency trading and even mining, albeit with a different time frame.
Broadly speaking, statistical arbitrage encompasses any strategy that employs statistical and econometric techniques to generate execution signals. Unsurprisingly, statistical arbitrage has become a significant force at both hedge funds and investment banks, where many proprietary operations revolve to varying extents around statistical arbitrage trading.
Now, returning to the cryptocurrency traders claiming to have capitalized on arbitrage opportunities, in our view, they have not truly realized arbitrage profits in the strictest sense of the term.
The definition of arbitrage stipulates that “arbitrage occurs when an investor simultaneously buys and sells an asset.” However, in the current landscape, with transaction verification on different blockchains, the speed of transactions is not “nearly simultaneous.” At best, there exists a 10-15 minute window of risk, which is considerably riskier in the crypto market compared to the stock market when adjusting for median volatilities.
While some crypto traders may have achieved profits in certain instances, they have exposed themselves to risks. These risks primarily include holding the currency during the time window between acquiring cryptocurrency “A” on exchange “X,” transferring it to exchange “Y,” and selling it there. Apart from market risk exposure, they have also taken on credit risk associated with different exchanges, as well as numerous operational risks. Their lack of awareness regarding these risks and their quantification has created the “illusion” of arbitrage profits. In reality, those claiming to have realized profits have been fortunate, and liquidity risk has not worked against them. Everybody appears astute in a bull market.
To enhance the likelihood of profiting from arbitrage opportunities in the crypto market, we suggest aiming for at least two out of the three types of arbitrage mentioned earlier: deterministic, statistical, and regulatory.
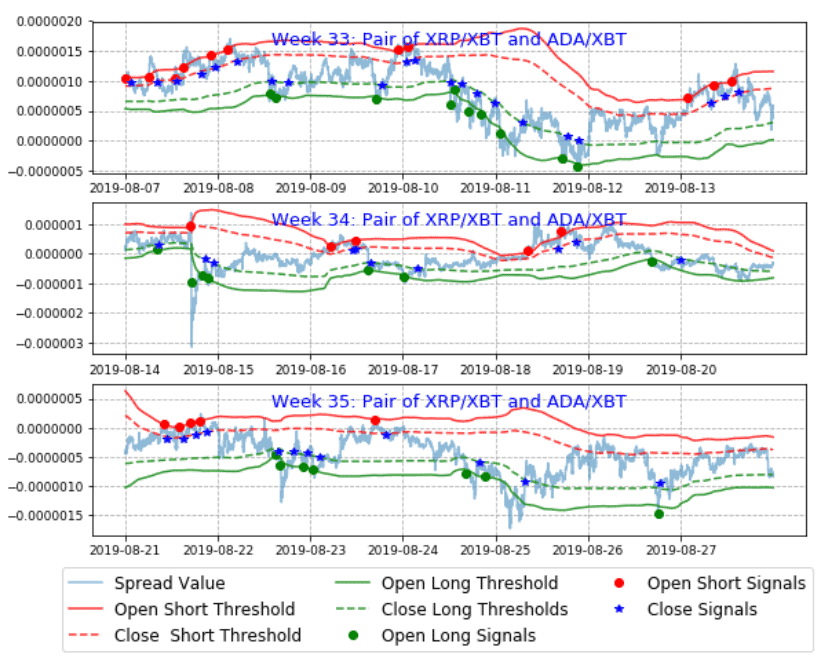
Using our custom code, we currently analyze transactional accounts in cryptocurrency exchange order books, which provide valuable market insights, alongside numerous other factors. Our code allows us to:
- Identify low-risk entry and exit points
- Detect outliers in price and volume data
- Identify high-probability changes in volatility
- Construct optimal portfolios of assets to hold within specific time frames
Our objective is to consistently outperform benchmarks on a risk-adjusted basis.
import ccxt
import numpy as np
from scipy.stats import zscore
# Connect to Binance exchange
def connect_to_exchange():
exchange = ccxt.binance({
'apiKey': 'YOUR_API_KEY',
'secret': 'YOUR_API_SECRET',
'enableRateLimit': True,
# Add additional configuration options if needed
})
return exchange
# Function to detect outliers in price and volume data
def detect_outliers(exchange, symbol):
# Fetch historical price and volume data
ohlcv_data = exchange.fetch_ohlcv(symbol, timeframe='1d', limit=100)
prices = np.array([ohlcv[4] for ohlcv in ohlcv_data])
volumes = np.array([ohlcv[5] for ohlcv in ohlcv_data])
# Calculate z-scores for prices and volumes
price_zscores = zscore(prices)
volume_zscores = zscore(volumes)
# Define threshold for outlier detection
price_threshold = 3.0 # Adjust as needed
volume_threshold = 3.0 # Adjust as needed
# Find indices of outliers
price_outliers = np.where(np.abs(price_zscores) > price_threshold)[0]
volume_outliers = np.where(np.abs(volume_zscores) > volume_threshold)[0]
# Print the detected outliers
print("Price outliers:", price_outliers)
print("Volume outliers:", volume_outliers)
# Main function to execute the trading strategy
def execute_trading_strategy():
exchange = connect_to_exchange()
symbol = 'BTC/USDT' # Replace with the symbol you want to analyze
# Call the detect_outliers function
detect_outliers(exchange, symbol)
# Entry point of the program
if __name__ == "__main__":
execute_trading_strategy()
In this example, the detect_outliers()
function fetches the historical price and volume data for a given symbol (e.g., ‘BTC/USDT’) from the Binance exchange using the fetch_ohlcv()
method. It then calculates the z-scores for both prices and volumes using the zscore()
function from the SciPy library.
You can adjust the price_threshold
and volume_threshold
variables to define the threshold for outlier detection. Higher thresholds will be more lenient, while lower thresholds will be more strict in identifying outliers. You may need to experiment and fine-tune these thresholds based on your specific requirements.
The function then identifies the indices of outliers by comparing the absolute values of z-scores with the defined thresholds. Finally, it prints the indices of the detected price and volume outliers.
Feel free to further customize and expand the code to suit your needs, such as incorporating additional outlier detection techniques or integrating it into your arbitrage trading strategy.
To ensure profitable cryptocurrency arbitrage, it is crucial to take a meticulous approach. Before investing any capital, it is recommended to thoroughly quantify all potential risks and conduct extensive out-of-sample tests. Furthermore, possessing domain expertise can greatly enhance your chances of success. If you lack professional trading experience, consider partnering with individuals who share your interest but have practical knowledge in financial engineering within real-world trading environments. Collaborating with such experts can provide valuable insights and increase the effectiveness of your arbitrage endeavors.
# Install required packages
install.packages(c("quantmod", "PerformanceAnalytics"))
# Load required libraries
library(quantmod)
library(PerformanceAnalytics)
# Set the start and end date for data retrieval
start_date <- as.Date("2022-01-01")
end_date <- Sys.Date()
# Define the symbols of the cryptocurrencies to be analyzed
symbol1 <- "BTC"
symbol2 <- "ETH"
# Download historical data for the two symbols
getSymbols(c(symbol1, symbol2), from = start_date, to = end_date, src = "yahoo")
# Create a merged data frame with adjusted closing prices for the two symbols
prices <- merge(Cl(get(symbol1)), Cl(get(symbol2)))
# Calculate the spread between the two symbols
spread <- prices[, 1] - prices[, 2]
# Implement a simple mean-reverting strategy with a threshold for entry and exit
entry_threshold <- 1
exit_threshold <- 0
# Initialize variables for tracking trade positions, P&L, and equity curve
position <- 0
pnl <- 0
equity <- 0
# Define risk management parameters
stop_loss <- -0.02
profit_target <- 0.02
# Backtest the strategy
for (i in 2:length(prices)) {
if (position == 0) {
if (spread[i - 1] > entry_threshold) {
# Enter a short position
position <- -1
entry_price <- spread[i]
} else if (spread[i - 1] < -entry_threshold) {
# Enter a long position
position <- 1
entry_price <- spread[i]
}
} else if (position == -1) {
if (spread[i - 1] < exit_threshold) {
# Exit the short position
position <- 0
exit_price <- spread[i]
pnl <- pnl + (entry_price - exit_price)
equity <- equity + pnl
pnl <- 0
}
} else if (position == 1) {
if (spread[i - 1] > -exit_threshold) {
# Exit the long position
position <- 0
exit_price <- spread[i]
pnl <- pnl + (exit_price - entry_price)
equity <- equity + pnl
pnl <- 0
}
}
# Apply risk management rules
if (position == -1 && pnl < stop_loss) {
# Exit the short position with stop loss
position <- 0
exit_price <- spread[i]
pnl <- pnl + (entry_price - exit_price)
equity <- equity + pnl
pnl <- 0
} else if (position == 1 && pnl > profit_target) {
# Exit the long position with profit target
position <- 0
exit_price <- spread[i]
pnl <- pnl + (exit_price - entry_price)
equity <- equity + pnl
pnl <- 0
}
}
# Calculate equity curve and performance metrics
equity_curve <- cumsum(c(0, equity))
returns <- Return.calculate(equity_curve)
metrics <- table.AnnualizedReturns(returns)
# Plot the spread and equity curve
par(mfrow = c(2, 1))
plot(prices, main = "Spread", ylab = "Price")
plot(equity_curve, main = "Equity Curve", ylab = "Equity")
# Print backtest results
cat("Backtest Results:\n")
print(metrics)
Please note that the above code provides a basic framework for backtesting a statistical arbitrage strategy and includes a simple mean-reverting approach. You may need to modify and customize the code according to your specific trading strategy and requirements. Additionally, ensure you have installed the necessary R packages (quantmod
and PerformanceAnalytics
) before running the code.