Unlocking the Power of Emotions: Deciphering the Fear & Greed Index
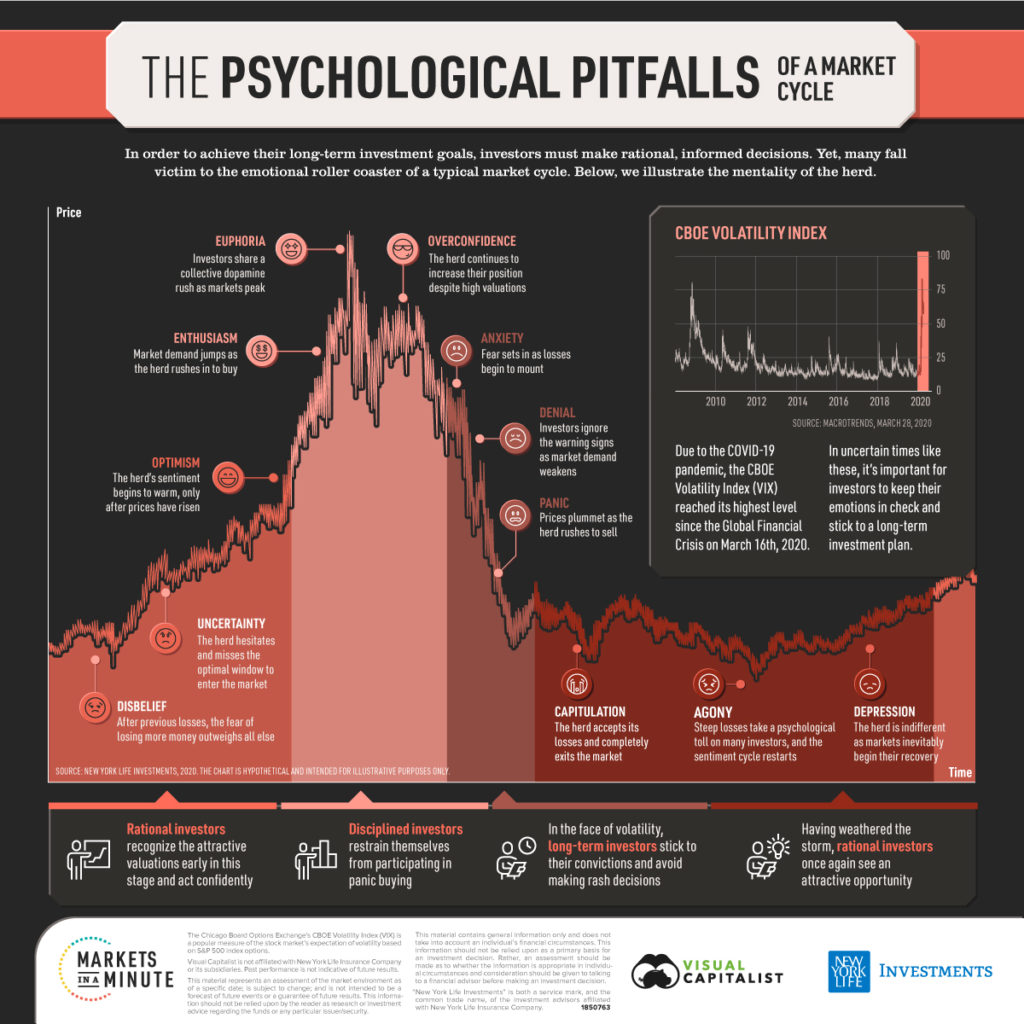
In the high-stakes world of financial markets, it’s not always fundamentals or technicals that move prices — it’s human emotion. Greed inflates bubbles; fear triggers crashes. Recognizing this, savvy investors look for tools that measure crowd psychology — and few are as insightful as CNN Business’s Fear & Greed Index.
This index is more than a sentiment snapshot. It’s a multi-factor gauge of emotional extremes, offering traders a way to time entries, exits, and risk exposure in volatile markets.
What Is the Fear & Greed Index?
CNN Business created the Fear & Greed Index to distill market sentiment into a single, intuitive number ranging from 0 (extreme fear) to 100 (extreme greed). This number is updated daily, weekly, monthly, and yearly, giving both short-term and macro-level views of investor behavior.
At its core lies a basic but powerful principle:
- Excessive fear often leads to undervalued stocks.
- Overwhelming greed can drive valuations far above their intrinsic worth.
The index, therefore, acts as a sentiment compass — alerting traders when the crowd might be on the wrong side of the trade.
Real-World Signals: Historical Context
The index’s historical extremes align eerily well with market inflection points:
- 2008 Financial Crisis: Dropped to 12 after Lehman Brothers collapsed, coinciding with the S&P 500’s plunge.
- September 2012: Surged past 90 as the Fed announced QE3, inflating equity prices globally.
- March 12, 2020: Hit a historic low of 2 amid COVID panic and Trump’s travel ban, right before one of the fastest bull runs in history.
- November 2020: Soared past 75 as vaccine optimism triggered aggressive risk-on behavior.
Time and again, the Fear & Greed Index has served as a powerful contrarian indicator.
Inside the Index: 7 Core Indicators
The index is a composite of seven sentiment-based signals, each scaled from 0 to 100. Here’s what powers the gauge:
- Stock Price Momentum: Tracks how far the S&P 500 is trading above or below its 125-day moving average.
- Stock Price Strength: Measures the ratio of stocks hitting 52-week highs vs. 52-week lows on the NYSE.
- Stock Price Breadth: Compares advancing vs. declining volume — are more shares gaining or losing ground?
- Put/Call Options Ratio: A contrarian gauge of trader fear vs. greed in the options market.
- Junk Bond Demand: Tight spreads = greed; wide spreads = fear.
- Market Volatility: Uses the VIX and its 50-day MA to gauge expected volatility.
- Safe Haven Demand: Assesses the relative performance of stocks vs. Treasuries.
Each component provides a different lens into market psychology, making the index a well-rounded sentiment signal.
Beyond Equities: Crypto’s Emotional Thermometer
Sentiment matters just as much in crypto — sometimes more. That’s why Alternative.me developed the Crypto Fear & Greed Index, tracking similar dynamics:
- Volatility
- Trading Volume
- Social Media Momentum
- BTC Dominance
- Google Trends data
While crypto’s version uses a different methodology, the aim is the same: measure how emotion drives price.
Build It Yourself: DIY Fear & Greed Index in Python
Want to go deeper? Here’s a blueprint to calculate your own Fear & Greed Index using real data and Python:
Step 1: Gather Market Data
You’ll need historical data for:
- S&P 500 (momentum)
- NYSE high/low stats (strength)
- Advancing/declining volume (breadth)
- Put/Call ratio
- Bond yield spreads
- VIX index
- Stock vs. Treasury returns
Step 2: Normalize to 0–100
Use Min-Max scaling:
normalized_value = (raw_value – min_value) / (max_value – min_value) * 100
Step 3: Average the Signals
fear_greed_index = (avg_momentum + avg_strength + avg_breadth + avg_options + avg_bond_demand + avg_volatility + avg_safe_haven) / 7
Step 4: Interpret the Score
- 0–24: Extreme Fear
- 25–44: Fear
- 45–55: Neutral
- 56–75: Greed
- 76–100: Extreme Greed
Step 5: Python Quick Example
import random
# Generate random normalized values for each indicator (0 to 100)
normalized_momentum = random.uniform(0, 100)
normalized_strength = random.uniform(0, 100)
normalized_breadth = random.uniform(0, 100)
normalized_options = random.uniform(0, 100)
normalized_bond_demand = random.uniform(0, 100)
normalized_volatility = random.uniform(0, 100)
normalized_safe_haven = random.uniform(0, 100)
# Calculate the average of normalized values
average_momentum = (normalized_momentum + normalized_strength + normalized_breadth + normalized_options + normalized_bond_demand + normalized_volatility + normalized_safe_haven) / 7
# Calculate the Fear & Greed Index
fear_greed_index = average_momentum
# Interpret the index
if fear_greed_index <= 24:
sentiment = "Extreme Fear"
elif 25 <= fear_greed_index <= 44:
sentiment = "Fear"
elif 45 <= fear_greed_index <= 55:
sentiment = "Neutral"
elif 56 <= fear_greed_index <= 75:
sentiment = "Greed"
else:
sentiment = "Extreme Greed"
print(f"Fear & Greed Index: {fear_greed_index} ({sentiment})")
This code generates random normalized values for each indicator, calculates the average, and interprets the Fear & Greed Index based on the score. In practice, you would replace the random data with real financial data to get meaningful results.
To create a Python script that fetches the Fear and Greed Index data from a reliable source, processes it, calculates the index, and plots it on a chart with automated updates, you can use libraries such as pandas
, yfinance
, matplotlib
, and schedule
. Here’s a step-by-step code example with explanations:
# Import necessary packages
import yfinance as yf
import pandas as pd
import matplotlib.pyplot as plt
import schedule
import time
# Define the function to fetch and process the Fear and Greed Index data
def fetch_process_fear_greed_index():
# Define the ticker symbol for the Fear and Greed Index
index_symbol = "^CNNFearGreed"
# Download historical Fear and Greed Index data from Yahoo Finance
df = yf.download(index_symbol, period="1y")
# Clean the data (remove missing values)
df.dropna(inplace=True)
# Calculate a simple moving average (SMA) for the index
window = 14 # Adjust the window size as needed
df['SMA'] = df['Close'].rolling(window=window).mean()
# Plot the Fear and Greed Index data and SMA
plt.figure(figsize=(12, 6))
plt.plot(df.index, df['Close'], label='Fear & Greed Index', alpha=0.7)
plt.plot(df.index, df['SMA'], label=f'{window}-Day SMA', color='orange')
plt.title('Fear & Greed Index and SMA')
plt.xlabel('Date')
plt.ylabel('Index Value')
plt.legend()
plt.grid(True)
plt.savefig('fear_greed_index_chart.png') # Save the chart as an image
plt.show()
# Schedule the function to run daily
schedule.every().day.at("09:00").do(fetch_process_fear_greed_index)
# Run the scheduled tasks
while True:
schedule.run_pending()
time.sleep(1)
We begin by importing the necessary libraries: yfinance
for downloading financial data, pandas
for data manipulation, matplotlib
for data visualization, and schedule
for task automation.
The fetch_process_fear_greed_index()
function is defined to retrieve and process the Fear and Greed Index. Within this function, we specify the appropriate ticker symbol representing the index.
Using yfinance
, we download historical data for the Fear and Greed Index. We then clean the dataset by removing any missing values and calculate a simple moving average (SMA) as a basic analytical example.
Next, we visualize the Fear and Greed Index alongside its SMA using matplotlib
. The resulting chart is saved as an image file (fear_greed_index_chart.png
) and displayed.
To automate execution, we schedule the fetch_process_fear_greed_index()
function to run daily at 9:00 AM using schedule.every().day.at("09:00").do(...)
.
Finally, the script enters an infinite loop (while True
) that continuously checks for scheduled tasks and executes them at the specified time. A one-second sleep interval between iterations helps minimize resource usage.
This script automatically retrieves the Fear & Greed Index data, applies a simple moving average (SMA), and generates a daily updated chart. You can easily customize parameters such as the SMA window or schedule time to better suit your trading workflow or analysis preferences.
Final Thoughts: Sentiment Is the Shadow of Every Trade
The Fear & Greed Index isn’t magic — it’s a mirror. It reflects the collective emotional state of investors, giving you a chance to zoom out from the noise and identify extremes.
Use it not as gospel, but as a counterweight to your own biases. In a world where narratives often overpower numbers, this index helps re-center your perspective.
Because in the end, successful investing isn’t just about predicting markets — it’s about managing emotion when markets go manic.